What is Big Data, Really?
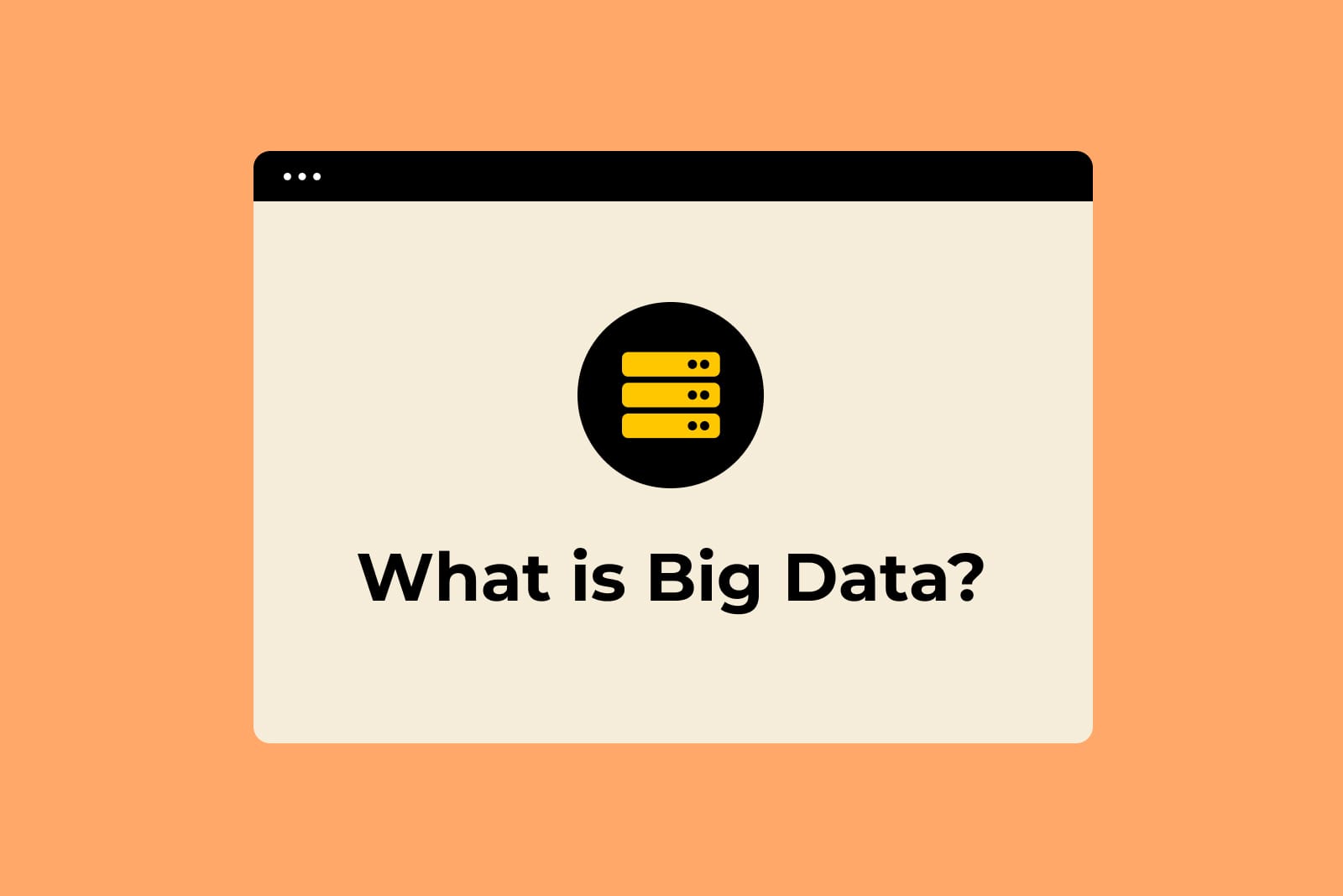
Everything you do* is data.
*online
Every time you click on a website? Data. That picture you just posted on social media? Data. Do you love watching your favorite shows and movies on your smart TV? It’s alllllll data! Everything is being captured and stored. And while it can feel invasive, all this data is — mostly — being used to make our lives a bit easier.
Businesses are tapping into this endless stream of data to make smarter decisions, create personalized experiences, and predict what you might like or need in ways that used to seem impossible. The exploding world of data is transforming whole industries, but the big challenge isn’t collecting that data. Trust me, there’s plenty of it. No, the real challenge is figuring out how to use it effectively (and ethically).
Data is everywhere, and understanding how to work with all of it is quickly becoming the key to professional success.
So, what is ‘Big Data’, really? Keep reading to learn about big data, how it works, and how some of the world’s biggest companies — like Netflix, Amazon, and Uber — are using it to create better customer experiences.
Bonus: We even break down three of the top jobs in big data (with six-figure salaries)! 🤩
Table of Contents
- What is Big Data?
- How Does Big Data Work?
- What are the five B’s of Big Data?
- The Benefits of Big Data (+ Examples)
- Finding a Job in Big Data
- Your Future in Big Data
What is Big Data?
Big data is exactly what it sounds like — a huge, overwhelming amount of information. But it’s not just about the size, it’s also about how we collect, manage, and extract meaning from these data sets. Think of it as the difference between your local library and the entire internet.
We live in a world where data is generated at incredible speeds, from social media posts to sensor readings on your smartphone… you know, the way your phone knows that you climbed five flights of stairs today and some of them were a struggle (I know, I know). With all this data, it’s simply too massive to be handled by traditional data tools.
Ultimately, there are three types of data: structured, unstructured, and semi-structured. Structured data is like a neatly organized spreadsheet — easy to read, easy to analyze. It includes numbers and text that fit neatly into rows and columns, like sales records or customer information.
Unstructured data, on the other hand, is the wild, wild west. Think videos, tweets, emails, or photos — tons of information that doesn’t fit neatly into boxes because of all the different channels and forms. Semi-structured data sits somewhere in between, like an email with a subject line, body text, and attachments. It has some structure but not enough to be fully categorized and cataloged.
As we mentioned, the real challenge with big data is figuring out how to make sense of it all and use it for good. But that’s also what makes it so valuable! By analyzing massive datasets, businesses can uncover hidden patterns, predict trends, and make smarter decisions. Simply put, it’s not just about collecting data, it’s what you do with it. So while the concept of big data may seem overwhelming, it’s also full of opportunities if you know how to harness its potential.
Related: Data Science 101: What it IS, What Data Scientists Do, and Real World Examples
How Does Big Data Work?
Big data works a lot in part due to advanced technology and the data scientists who are behind the scenes doing the work. And while it might not seem glamorous at first look, the professionals at Harvard Business School named data scientists the sexiest job of the 21st century. The combination of math, computer engineering, and AI doesn’t necessarily scream sex appeal to me, but the fact that big data helps companies boost revenue by 8% definitely does!
Let’s get into it.
Step 1. Data Collection
The first step is gathering data (duh?). Data can come from pretty much anywhere — social media, sensors in our cars, customer transactions, or even your smart TV. The goal is to pull in all sorts of information from structured data (like lists and numbers) to unstructured data (like social media posts or photos). Depending on the source, data scientists rely on a mix of tools for extraction. For example, SQL queries are ideal for databases while Python scripts are pretty universal with their ability to extract data from databases, APIs, websites, and CSV files.
Related: 20+ Ways to Learn SQL Online (For Free!)
Step 2. Data Storage
Now that you’ve got a methodology established to collect all this data, it needs a place to live. Traditional databases can’t handle the volume, so you’ll see data scientists use big data technologies (like Apache Hadoop) or cloud storage, which are designed for massive amounts of information. Importantly, these systems can scale as your data stockpile grows, so you never run out of room (hint: you’ll never have less data).
Step 3. Data Cleaning
Here comes the not-so-glamorous part: cleaning. Data from the exact same source can still come in dozens of different formats — text (TXT, HTML, XML), numeric, multimedia (JPEG, PNG, MP3, MP4), tabular data (XML, Excel), and more. Big data also usually contains duplicates, errors, and definitely irrelevant bits. Read: it’s messy and inconsistent.
So, it needs to be cleaned to get rid of any errors while ETL (extraction, transform, load) jobs “combine data from multiple sources into a single, consistent data set” for storage. This makes sure the data that’s left is useful and accurate — because messy data often translates to inaccurate insights. And data scientists (and the businesses they work with) definitely don’t want that.
Step 4. Data Analysis
Once the data is organized and cleaned, it’s time to analyze it. This is where the “fun” — if you’re into that kind of thing — happens. With the right tools, like machine learning algorithms or statistical models, data scientists uncover patterns, trends, and any unusual relationships in the data. It’s like finding out that your best sales days are when it’s raining — something you’d (probably) never guess by looking at the data manually.
Step 5. Visualization
The point of big data is to use these insights to make smarter business decisions. Data scientists need to present the findings in a way that’s easy to understand. Data visualization is the representation of data through visual graphics — charts, graphs, maps, dashboards, etc., and yes, that includes PowerPoint. Those graphs, charts, and dashboards make it possible for stakeholders (who likely have no idea about the data process) to see these trends quickly, making it a lot easier for them to act on these insights.
Related: What is a Data Visualization Designer?
What are the five Vs of Big Data?
When people talk about the five Vs of Big Data, they’re really describing the key characteristics that make big data… well, big.
Volume
It’s probably the first thing that comes to mind when you think about big data. It’s all about how much data we’re dealing with. Forget megabytes (MB). We’re dealing with terabytes (TB), petabytes, and beyond. For reference, 1 TB = 1,000 gigabytes (GB) = 1,000,000 MB. For example, Facebook users upload over 350 million photos every day. That’s tons of information to store, manage and analyze. The sheer amount is overwhelming, but again — that’s part of what makes it so valuable.
Velocity
Velocity is the speed at which data is created and the speed required for processing. This actually has less to do with the amount of data, and more to do with how fast it’s received. For example, think of the data flooding in from sensors in self-driving cars. Every second, these vehicles are gathering real-time data about the road, the weather, and traffic. On a car-by-car basis (ignoring for the moment that all of these cars can transmit their data to make better cars), this kind of fast-moving data needs to be processed in real-time so instant decisions can be made — like when to stop or turn. This is why velocity is a big deal: it’s not enough to have data — you need it to be actionable immediately.
Variety
Variety is the spice of life, right? Big data isn’t just one kind of data. It’s massively diverse — text, images, videos, sound, and even sensor data like in our car example and social media posts count as big data. Think about how your YouTube recommends videos based on both structured data (like what you’ve watched or clicked off of) and unstructured data (like the comments you leave). Variety is a key component of big data because it means you’re dealing with a mix of formats that need to be parsed, understood, and analyzed, so their insights make sense in a bigger context.
Veracity
Veracity refers to the trustworthiness or quality of the data. Just because there’s a lot of data doesn’t mean it’s useful. Imagine receiving thousands of customer reviews for a product, but a lot of them come from bots. You need to make sure the data you’re working with is accurate, reliable, and consistent — otherwise, your insights will be all over the place and plain old inaccurate. This is where data cleaning comes into play, which helps to separate the noise from the valuable information.
Value
Last, but not least, we have value. All that data — if you can store, process, and trust it — should offer some value. It’s not just about having a bunch of numbers or text floating around. It’s about getting insights that can help a business make better decisions. For example, big retailers like Walmart use big data to predict shopping trends, optimize their inventory, and improve customer satisfaction. If the data doesn’t lead to actionable insights or improvements, what’s the point?!
The Benefits of Big Data (+ Examples)
Big data isn’t just a buzzword, it’s become an integral part of how businesses, organizations, and even governments handle their operations. I mean, there’s a reason why companies are paying data scientists and big data developers six-figure salaries. These jobs are in demand and typically offer tons of benefits with their work.
Some of the benefits that companies get from leveraging big data include:
- Smarter decision-making. By analyzing large amounts of data, businesses can uncover trends and patterns that would otherwise go unnoticed. For example, Target uses shopping patterns to predict customer preferences, helping them tailor promotions and use marketing techniques like push notifications more effectively.
- Better customer experiences. Big data helps organizations understand their customers better, helping make their experience more personal. Spotify analyzes your listening habits to create customized playlists in a wide variety of formats (Release Radar and Daylists, for example) so they can keep you online with music they think you’ll like.
- New product development. Companies can use big data to spot gaps in the market or track evolving trends. A company like Nike can use customer feedback and performance data to design new, improved athletic gear, a benefit if no one else is developing in that space yet.
- Predictive analytics. Using historical data, businesses can forecast trends and behaviors. Netflix notably uses predictive analytics for two big tasks: recommending what you might watch next and predicting the next big original series.
- Cost savings. Big data helps companies pinpoint their inefficiencies, which can help reduce costs. UPS uses real-time data to optimize delivery routes, which helps save millions on fuel costs while reducing their carbon footprint.
- Competitive edge. Big data can help companies get ahead of the curve. Look at Amazon — they track customer behaviors across multiple platforms to refine their inventory and pricing strategies so they can stay ahead of competitors and keep their customers coming back.
- Improved risk management. Companies use big data to help identify potential risks before they become problems. Banks and insurance companies can use big data to detect fraud patterns while businesses can use it to spot operational risks.
Finding a Job in Big Data
Imagine you’ve just been handed an enormous stack of puzzle pieces. Each piece is a bit of information — numbers, text, images, videos — stuff that, on its own, doesn’t make much sense. But when you figure out how to arrange them…you’ll reveal the big picture. That’s essentially what working in big data feels like — using your skills to make sense of complex, messy information to solve real-world problems.
If you’re thinking about pursuing a career in big data, here are three of the most common jobs you could aim for:
Data Scientist
Data scientists take huge amounts of raw data and use algorithms, statistical models, and machine learning techniques to extract insights and make predictions. They find patterns that others might miss and turn them into actionable knowledge. You’ll do more than analyze data. You’ll build complex models that predict future outcomes, discover patterns, or make recommendations.
For example, if you were working as a data scientist at Google, you’d use large amounts of data to improve their search algorithm. This means developing machine learning models that can more accurately predict which search results will be most relevant to the searcher.
Skills Needed:
- Programming languages (Python, R)
- Big data technologies (Apache Hadoop and Apache Spark)
- Database management and tools (SQL, NoSQL, Microsoft Excel)
- Machine learning techniques (Linear regression, logistic regression, decision trees. etc.)
- Data visualization
Data Engineer
As a data engineer, you’ll design and build systems and infrastructure that allow data scientists and analysts to do their jobs. It’s like drawing the plans for a house — you have to make sure one room flows into the next. The same goes for data, and you’ll make sure the data flows smoothly from one place to another so that it’s securely stored and subsequently accessible.
If you worked as a data engineer at Uber, your job would include making sure that ride data from thousands of trips are collected in real time and stored. Without a solid data engineering team, it would make it nearly impossible for Uber to try to analyze data to improve routes or predict rider demand.
Skills Needed:
- Programming languages (Python, Java, Scala)
- Data warehousing
- Database management and tools (SQL, NoSQL, Microsoft Excel)
- Data pipeline tools (Apache Kafka, Airflow)
- Cloud computing (AWS, Azure)
Machine Learning Engineer
Machine learning engineers take the models that data scientists create and turn them into functioning systems. If you worked at Amazon, you’d constantly tweak the algorithms behind their recommendation systems. Every time someone receives a “Customers who bought this also bought…” suggestion, that would be the result of your work and a machine learning model that was fine-tuned over countless hours of data analysis and engineering.
Skills Needed:
- Programming languages (Python, C++, Java)
- Machine learning techniques (Linear regression, logistic regression, decision trees. etc.)
- Machine learning frameworks (TensorFlow, PyTorch)
- Data engineering (data extraction, ETL processes)
- Cloud computing
Your Future in Big Data
Have you ever heard the acronym C.R.E.A.M. (Cash Rules Everything Around Me)? I’m starting to think it should be D.R.E.A.M. for Data Rules Everything Around Me. Big data transforms how industries — entertainment, healthcare, finance, and more — operate, helping them make smarter decisions and improve customer experiences. From predicting the next blockbuster movie to optimizing supply chains, big data shapes how we live and work. And as companies get better at using the power of big data, they’re not just adapting to the future — they’re helping create it.
The demand for data professionals and data-driven insights is growing fast, and you might find yourself wanting to jump on the big data train. If you don’t know where to start, start here — with the Skillcrush Break Into Tech program. It’s the first step to learning the fundamentals of web development that will help you prepare for a successful future in big data.